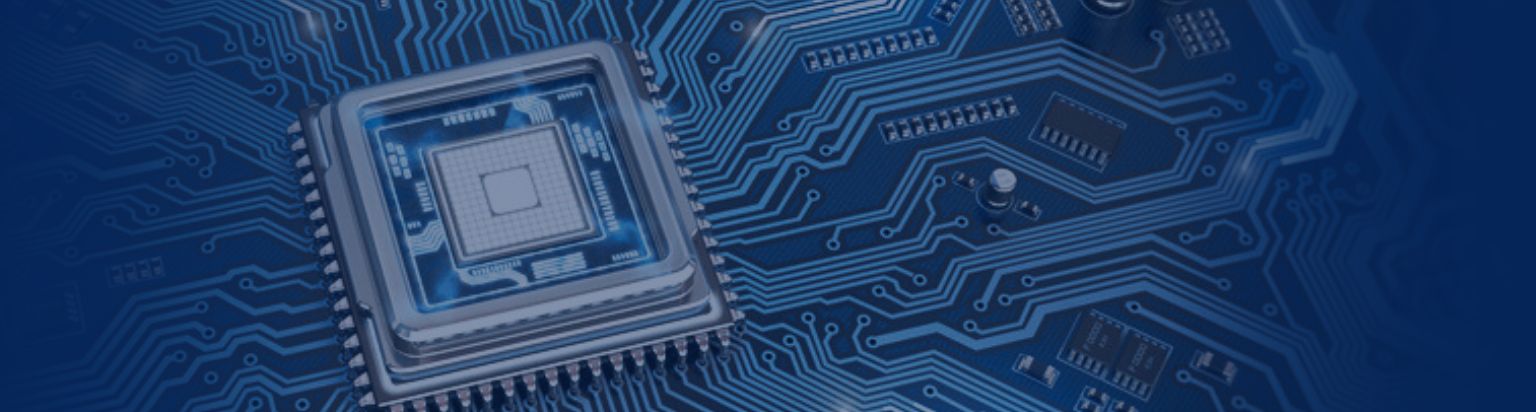
Importance of VLSI Design Verification and its Methodologies
In the dynamic world of VLSI (Very Large-Scale Integration), the demand for innovative products is higher than ever. The journey
In the dynamic world of VLSI (Very Large-Scale Integration), the demand for innovative products is higher than ever. The journey
In recent years, Field Programmable Gate Arrays (FPGAs) have emerged as a viable technology for High-Performance Computing (HPC), thanks to
The development of VLSI technology has opened up new possibilities in the field of microelectronics. The landscape of electronic systems
Designing a chip is a complex and multi-step process that encompasses various stages, right from initial system specifications…
Minimizing power consumption is a major factor that contributes to the modern-day development of IC designs, especially in the consumer …
Due to their excellent performance and versatility, FPGAs (Field Programmable Gate Arrays) appeal to a wide spectrum of businesses. Also, it has the…
Propelled into existence riding on low NRE (Non-recurring Engineering) cost, the FPGAs began as an alternative to ASIC until the customer required…
Copyright © 2023 Softnautics (a MosChip company) |
All Rights Reserved
Cookie | Duration | Description |
---|---|---|
cookielawinfo-checkbox-analytics | 11 months | This cookie is set by GDPR Cookie Consent plugin. The cookie is used to store the user consent for the cookies in the category "Analytics". |
cookielawinfo-checkbox-functional | 11 months | The cookie is set by GDPR cookie consent to record the user consent for the cookies in the category "Functional". |
cookielawinfo-checkbox-necessary | 11 months | This cookie is set by GDPR Cookie Consent plugin. The cookies is used to store the user consent for the cookies in the category "Necessary". |
cookielawinfo-checkbox-others | 11 months | This cookie is set by GDPR Cookie Consent plugin. The cookie is used to store the user consent for the cookies in the category "Other. |
cookielawinfo-checkbox-performance | 11 months | This cookie is set by GDPR Cookie Consent plugin. The cookie is used to store the user consent for the cookies in the category "Performance". |
viewed_cookie_policy | 11 months | The cookie is set by the GDPR Cookie Consent plugin and is used to store whether or not user has consented to the use of cookies. It does not store any personal data. |